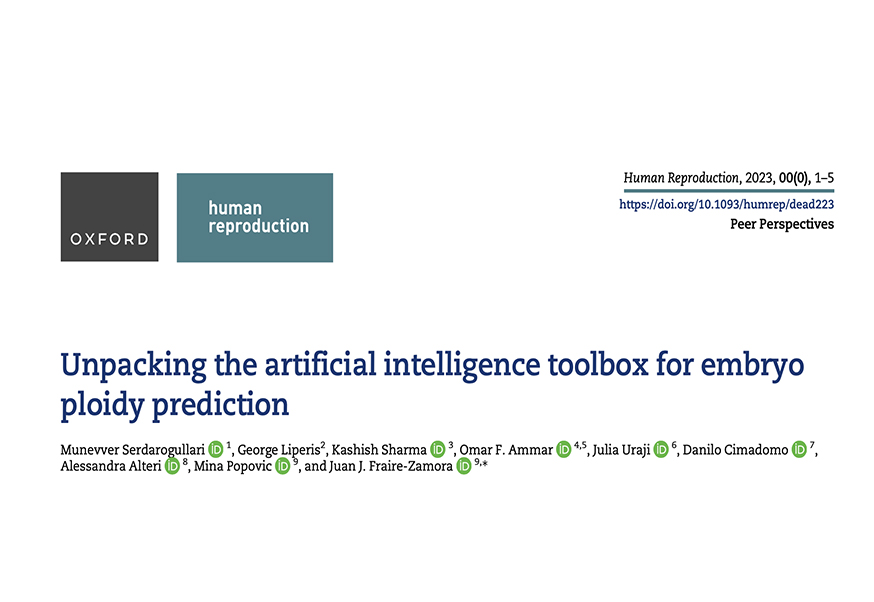
Munevver Serdarogullari, George Liperis, Kashish Sharma, Omar F. Ammar, Julia Uraji, Danilo Cimadomo, Alessandra Alteri, Mina Popovic, and Juan J. Fraire-Zamora
Human Reproduction, Volume 38, Issue 12, December 2023, Pages 2538–2542, https://doi.org/10.1093/humrep/dead223
ABSTRACT
Bamford et al., (2023) evaluated 12 ML models for blastocyst ploidy prediction, finding logistic regression surpassed more complex algorithms.
The role of age in ML models
- Bamford’s model was trained in a population of older oocyte providers
- Medical datasets have inherent biases based on the population sampled
- ML model generalisation across ages is questioned
- The role of oocyte age in ML depends on the statistical unit (population vs. cohort)
From dataset imbalances to clinical decisions
- Dataset imbalances impact ML clinical generalisation
- Interdisciplinary teamwork is key for ML-based decision making
- Simple, interpretable models can outperform complex ones
- Clinicians must grasp ML methods for successful IVF integration
Using ML to revitalise the ‘classics’
• Bamford’s model using clinical variables and embryo morphology is a valid alternative for clinics without timelapse technology
• The goal of ML-based embryo selection should be to predict a live birth
• ML-based embryo assessment must be used as a tool for clinical decisions without replacing professionals
The May ESHRE Journal Club discussion focused on a study by Bamford et al. (2023), ML models for predicting ploidy status of embryos, IVF dataset characteristics and imbalances, and the use of ML-based embryo assessment as a tool for clinical decisions. ML, machine learning.